Critical Machine Learning Studies: An Interview with Fabian Offert and Rita Raley
by
Fabian Offert and Rita Raley (UC Santa Barbara) spoke to UCHRI Research Grants Program Director, Sara Černe, about their UCHRI-funded Critical Machine Learning Studies faculty working group, which planted the seeds for new collaborations, including Offert’s international research project AI Forensics, funded by the Volkswagen Foundation.
“Artificial intelligence, to quote Phil Agre, “is philosophy underneath”—in other words, all the important questions that emerge from today’s machine learning models are questions that the humanities have been addressing for centuries.”
Tell us about your project and how it complicates the discussions of AI we hear in the media.
“Critical Machine Learning Studies” aims to articulate, from within the humanities, a technically specific and situated paradigm for engaging machine learning. And our rationale for choosing to focus on “machine learning”—the technical backbone of “artificial intelligence”—and not “artificial intelligence” points directly to what we see as the main contribution of the project.
Much of the journalistic coverage of AI (and even some academic work) treats it as a homogenous cultural technique, or generalizes about a technocultural condition or situation. There are good practical and rhetorical reasons why this would be the case: Even with all of the news coverage, this domain of research is still new for many, and a certain level of generality does make it possible for more people to participate in the conversation, which is especially important for policy and politics. You can use that language and presume that people will have an intuitive understanding of what it is they’re asked to think about. And while “AI” is reductive shorthand, and arguably now just a siren call for attention and investment, it also allows for some referential consistency over time, which is especially necessary because of the rapid pace of both research and practical implementations. Even ChatGPT—although still discussed on college campuses as the next big thing—seems already to belong to the past in the context of technical research, but it will seem especially so in a year, if not a few months.
One could certainly try to set policy for, and ask philosophical and political questions about, large language models in general. But such analyses would necessarily need to generalize because models are always evolving. This would be an unremarkable observation except that there is now quite a lot of empirical work demonstrating that scaling size and training compute have a direct effect on model capabilities and behaviors. In other words, whatever we might know or discover about a particular model will likely become obsolete once it is scaled up or down, even if some of the techniques of sampling and decoding remain relatively consistent. Epistemological questions raised by one version of a model might not be raised by later versions. As machine learning models evolve, in other words, so do their social, political, and philosophical ramifications.
Researchers will sometimes use the phrase “interpretable window” when discussing a study of model behavior, which does nicely capture the critical position available to us: on the outside, peering in at a complex system and trying to guess how it will evolve given its initial conditions and input parameters. It also helpfully delimits and circumscribes the space of investigation: We have one window onto one model in one moment in time (if run through an API there are further variables). This suggests that our collective deliberations about harms, misuses, and errors need to be better grounded in analyses of specific models at specific points in time (indeed, the technical literature itself uses the term “snapshot”). And it also suggests that academic work should do the same, to yoke critical and theoretical questions to actual machine learning systems.
The intent of our project then was to try to develop, again from within the humanities, a critical framework that focuses on such “snapshots,” on specific machine learning architectures turned into idiosyncratic models of, for instance, literary or visual culture. The methodology we are working toward—it seems appropriate that it would be always in process—establishes a critical relationship to inherently dynamic objects. More specifically, the methodological intervention takes rapid differentiation—in scale and domain—into account.
Not only does our project seek to challenge the conception of “artificial intelligence” as a uniform technology but, perhaps more provocatively, “Critical Machine Learning Studies” also presents a challenge to “Critical AI Studies.” Before identifying the methodological and conceptual difference between “Critical ML” and “Critical AI” as we understand it, we do need to add an important caveat. The language of “critical AI” is popping up everywhere, in research programs and centers, publications, and courses (including our own), and it is too soon to pronounce definitively on the parameters of the field, which is after all in formation. Nonetheless, there is enough of an archive now to support the observation that “critical AI” has coalesced around a set of methods that are at their core variations of speculative close reading. In other words, “critical AI” foregrounds interpretations of “artificial intelligence” as a media discourse, rather than interpretations of the actual techniques, models, and systems informing that discourse. Just as “AI” suffers from reductiveness but also has its pragmatic uses, so too does “Critical AI” implicitly extend an invitation to think in general terms across disciplines about issues such as labor, infrastructure, environment, and the transmutation of culture and life itself into training data. Certainly, there is an urgent need for both micro- and macro-level work on the AI industry as such, and this truly is a moment for leveraging all available tools. But in our view the most productive critical work will not pose research questions about machine learning at a level of abstraction as to be almost wholly severed from its material ground.
“Not only does our project seek to challenge the conception of ‘artificial intelligence’ as a uniform technology, but, perhaps more provocatively, ‘Critical Machine Learning Studies’ also presents a challenge to ‘Critical AI Studies.'”
What do you see as the main contribution of the humanities to machine learning studies and how might humanistic methods inform the field?
We would like to politely refuse the terms of the question here as it implies that the humanities is coming to machine learning from the outside. Artificial intelligence, to quote Phil Agre, “is philosophy underneath”—in other words, all the important questions that emerge from today’s machine learning models are questions that the humanities have been addressing for centuries. For instance, how can knowledge about the world be represented efficiently, and what is the exact difference between a representation of a thing and the thing itself? Entire subfields of computer science like interpretable machine learning or representation learning are essentially concerned with these and similar questions.
Research in visual artificial intelligence can helpfully illustrate the point. Knowledge about the visual world that is learned by a machine learning model is not “conceptual;” instead, it is “entangled,” meaning that the same part of a model, the same “neuron” in technical terms, is responsible for doing a host of different things. This leads to all kinds of technical roadblocks, for instance the issue of “shortcut learning,” where models will pick up ways to solve a task that are efficient but defy the rules of the task. The famous instance of this is a classifier that is supposed to detect cows but fails on a photo of a cow on the beach because it has learned that a “cow” is an object in front of a green pasture, rather than a black and white animal of a certain size. There are countless other examples that demonstrate, over and over again, how the similarities and differences between human and machine ways of seeing the world point to philosophical issues with which the humanities have long been concerned.
“How can we make our critiques better; and how can we make them even more thorough, by tying them back to actual technical developments? If we concretize arguments about risks in practice and experiment, how much more persuasive could they be?”
So, rather than thinking about a post-hoc contribution of the capital H humanities to artificial intelligence, for this project we set out to think about the translation between concrete technical developments and concrete philosophical problems, as well as concrete disciplinary approaches within the humanities that might address them. To be clear, we are not rejecting the idea that the humanities should prominently and publicly voice general concerns about artificial intelligence—for instance related to its foundations in eugenics, the industry’s exploitation of workers, or its role in enabling new forms of political and social surveillance. We are also not interested in making the models “better” in the sense of optimizing them, or in “helping out” computer science. We are instead asking: How can we make our critiques better, and how can we make them even more thorough, by tying them back to actual technical developments? If we concretize arguments about risks in practice and experiment, how much more persuasive could they be?
We could also turn the question around and ask how critical machine learning studies might inform the humanities. Here we would point to the necessarily collaborative aspect of research practices, whether formally supported by institutions or the result of informal, temporary connections facilitated by open source models and repositories. The turn toward collaborative and applied research in the humanities of course happened well before AI/ML in its current manifestation, but arguably the expansion of authorship simply means the expansion of IP rather than a paradigm shift, and it would be interesting to see what might change if our disciplines genuinely prioritized sharing and cooperation.
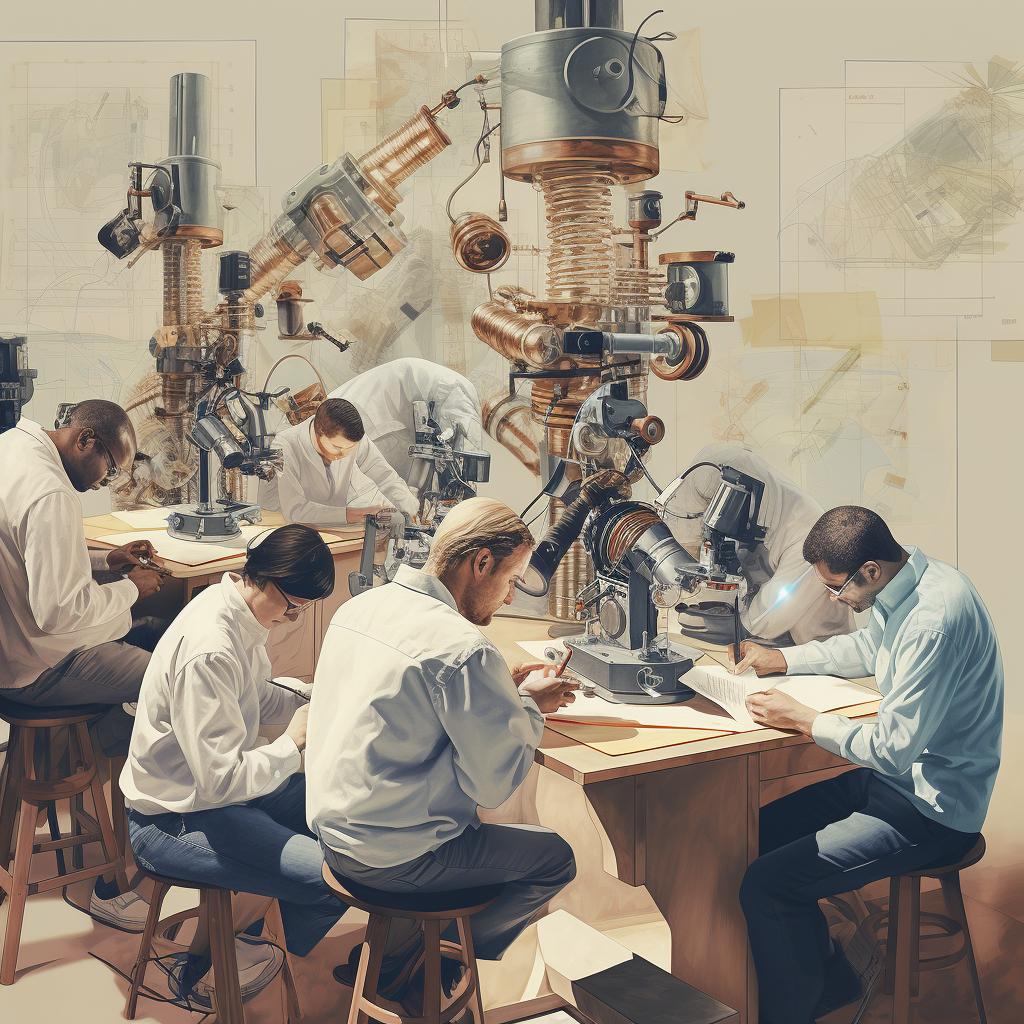
How do you approach critical ML studies in a pedagogical context? What new horizons have you seen open up for students as they explore multiple literacies in your classrooms?
It is our firm belief that the classroom has to become something like a counterweight to popular (mis)information about artificial intelligence. Up until the moment that artificial intelligence started to be discussed in the media as a matter of fact rather than speculative fiction—OpenAI’s chat application initiated this shift—humanities classes almost necessarily had to have recourse to cultural representations to start the conversation. There is of course a rich literary, cinematic, and televisual history of artificial beings, so one could draw on these texts (especially those that are more recent such as Her and Ex Machina) as conceptual frames. So in the past if students were asked to draw a picture of artificial intelligence on the first day, inevitably you would see a lot of robots. But now students come to class with concrete ideas and concrete fears about these systems, and the same exercise leads to visual approximations of neural networks, and the occasional black box. Students might not have played around with image generators or with AI-based filters on their phones, and they might even not have tried ChatGPT, but certainly they have heard about these things, and all together they can come up with a fairly comprehensive list of implementations in different industries. Even if they think they don’t have first-hand experience of AI, it doesn’t take long for them to realize that they do, and they also have a clear sense of the harms and risks, especially with regard to discrimination and the automation of the workforce.
An important question for students then, but really for everyone, is what is to be done. Refusal and resistance are available paths forward, but we each try in our own way to make the conversation concrete. One example would be a discussion of the anti-AI makeup that made the rounds on social media a few years ago; this lends itself to a conversation about technical specifics because it only works with a narrow class of facial recognition models. Another popular exercise is to close read the infamous “gay face” paper (2018) that claimed to be able to infer sexual orientation from facial features. We read the paper and collect all the implicit assumptions it makes, both about human sexuality and about the capabilities of artificial intelligence. In direct contrast with the media narrative of the impenetrable black box of artificial intelligence, students see many of the flaws immediately just by following along with the technical literature (which is often complicated only because of jargon, not because of the complexity of ideas). The students, in other words, are eager to learn ways to look behind the scenes, and our pedagogies take this into account.
“It is our firm belief that the classroom has to become something like a counterweight to popular (mis)information about artificial intelligence. […] The students are eager to learn ways to look behind the scenes, and our pedagogies take this into account.”
Another pedagogic exercise is to ask students to find an object that can be the basis for a research report as devised for the Transliteracies project, which was also as it happens a UC-system working group, one that aimed to explore the sociocultural and technological aspects of online reading. The report template works particularly well for courses in AI/machine learning, not only because the sections asking for “research context” and “technical analysis” help to concretize the issues raised in discussion, but also because the requirement that students provide a “synopsis of main technical specifications, methods, or approaches” asks them to translate for themselves what is again often presented as mysterious and unexplainable. This exercise also allows them to explore their respective disciplinary interests: Recent highlights include reports on a model used for drug development, on a smart port, and on an artist working with image generation.
This is the segue to the last thing to note, which is that machine learning’s entry into the humanities classroom builds upon already-extensive curricula devoted to creative uses of computational media. For courses in media arts and history, electronic literature, technologies of writing, game studies, and the post-literary, generative AI is the logical next-step.
What is the main impact of your work, how has UCHRI grant funding helped you achieve it, and how has the project evolved since?
For this grant project, we were able to leverage a range of specializations and forms of expertise to help sketch the parameters of our collective methodological intervention. Both of us have worked on very different kinds of models (Rita on large language models [GPT-2], Fabian on large visual models), and other members of the group have brought their unique research perspectives to the table. Our group drew from the Digital Humanities, Film and Media Studies, Science and Technology Studies, as well as our respective home departments of German and English, and we were able to put individual research emphases on computer graphics, nanotechnology, science fiction, and surveillance into dialogue with machine learning. Throughout, we sought intersections and moments of synthesis via collective analysis of technical papers. Goodfellow et al.’s foundational paper on the Generative Adversarial Network (2014), for example, gave rise to an extended conversation about adversariality and about the metaphors and fables used to describe model functioning.
It was wonderfully productive to discuss these ideas with colleagues during the grant period; the UC system is host to a fantastic group of researchers, and the UCHRI grant allowed us to tap into this shared resource in a strategic and productive way. It has given us the means to lay the foundation for our collective work toward establishing “critical ML studies” as a humanities response to the ongoing differentiation of model architectures, applications, and implementations, and we are grateful for the support.
Banner image generated by Midjourney using the prompt “A painting of a high-performance GPU graphics card by Max Ernst.”